申博直营网博彩平台客服服务质量_Flink奉行经由与源码分析
本文转载自微信公众号「大数据左右手」,作家王了个博。转载本文请臆想大数据左右手公众号。
Flink主要组件(1) 限度一个诈骗步骤奉行的主程度,也即是说,每个诈骗步骤 皆会被一个不同的Jobmanager所限度奉行
(2) Jobmanager会先吸收到要奉行的诈骗步骤,这个诈骗步骤会包括:功课图( Job Graph)、逻辑数据流图( ogical dataflow graph)和打包了通盘的类、库和其它资源的JAR包。
(3) Jobmanager会把 Jobgraph蜕变成一个物理层面的 数据流图,这个图被叫作念 “奉行图”(Executiongraph),包含了通盘不错并发奉行的任务。Job Manager会向资源处分器( Resourcemanager)请求奉行任务必要的资源,也即是 任务处分器(Taskmanager)上的插槽slot。一朝它得回到了裕如的资源,就会将奉行图分发到信得过运行它们的 Taskmanager上。而在运行过程中Jobmanagera会厚爱通盘需要中央配合的操作,比如说查验点(checkpoints)的配合。
任务处分器(Taskmanager)(1) Flink中的使命程度。频繁在 Flink中会有多个 Taskmanageria运行, 每个 Taskmanageri皆包含了一定数目的插槽( slots)。插槽的数目规模了Taskmanageri或者奉行的任务数目。
(2) 启动之后, Taskmanager会向资源处分器注册它的插槽;收到资源处分器的提醒后, Taskmanageri就会将一个或者多个插槽提供给Jobmanageri调用。Jobmanager就不错向插槽分派任务( tasks)来奉行了。
(3) 在奉行过程中, 一个 Taskmanagera不错跟其它运行归拢诈骗步骤的Taskmanager交换数据。
资源处分器(Resource Manager)(1) 主要厚爱处分任务处分器( Task Manager)的 插槽(slot)Taskmanger插槽是 Flink中界说的处理资源单位。
(2) Flink 为不同的环境和资源处分用具提供了不同资源处分器,比如YARNMesos、K8s,以及 standalone部署。
(3) 当 Jobmanager苦求插槽资源时, Resourcemanager会将有优游插槽的Taskmanager?分派给Jobmanager。要是 Resourcemanagery莫得裕如的插槽来自在 Jobmanager的请求, 它还不错向资源提供平台发起会话,以提供启动 Taskmanager程度的容器。
分发器(Dispatcher)(1) 不错跨功课运行,它为诈骗提交提供了REST接口。
(2)当一个诈骗被提交奉行时,分发器就会启动并将诈骗交代给Jobmanage
(3) Dispatcher他会启动一个 WebUi,用来浮浅地 展示和监控功课奉行的信息。
任务提交经由a. Flink任务提交后,Client向HDFS上传Flink的Jar包和竖立
b. 随后向 Yarn ResourceManager提交任务ResourceManager分派 Container资源并奉告对应的NodeManager启动
c. ApplicationMaster,ApplicationMaster 启动后加载Flink的Jar包和竖立构建环境
d. 然后启动JobManager , 之后ApplicationMaster 向ResourceManager 苦求资源启动TaskManager
博彩平台客服服务质量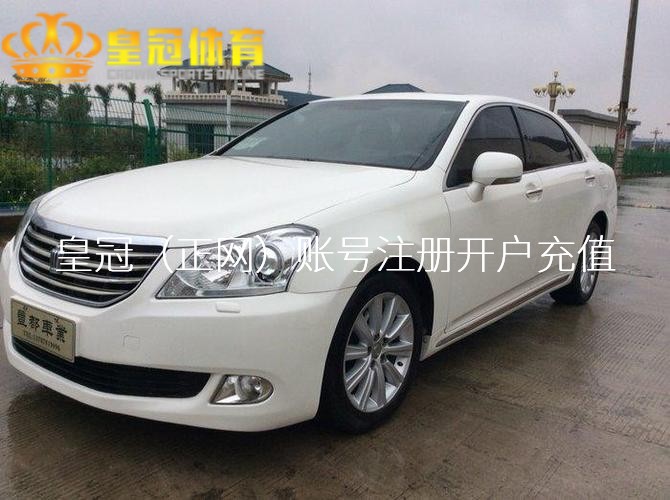
e. ResourceManager 分派 Container 资源后 , 由ApplicationMaster奉告资源所在节点的NodeManager启动TaskManager
皇冠体育搭建f. NodeManager 加载 Flink 的 Jar 包和竖立构建环境并启动 TaskManager
g. TaskManager 启动后向 JobManager 发送心跳包,并恭候 JobManager 向其分派任务。
源码分析--集群启动 JobManager 启动分析 JobManager 的里面包含相等贫苦的三大组件 WebMonitorEndpoint ResourceManager Dispatcher 进口,启动主类:StandaloneSessionClusterEntrypoint// 6868在线入 口 StandaloneSessionClusterEntrypoint.main() ClusterEntrypoint.runClusterEntrypoint(entrypoint); clusterEntrypoint.startCluster(); runCluster(configuration, pluginManager); // 第一步:运行化各式工作 /** * 运行化了 主节点对外提供工作的期间所需要的 三大中枢组件启动时所需要的基础工作 * 运行化工作,如 JobManager 的 Akka RPC 工作,HA 工作,心跳查验工作,metric service * 这些工作皆是 Master 节点要使用到的一些工作 * 1、commonRpcService: 基于 Akka 的 RpcService 已毕。RPC 工作启动 Akka 参与者来吸收从 RpcGateway 调用 RPC * 2、haServices: 提供对高可用性所需的通盘工作的拜谒注册,散播式计数器和引导东说念主选举 * 3、blobServer: 厚爱侦听传入的请求生成线程来处理这些请求。它还厚爱创建要存储的目次结构 blob 或临时缓存它们 * 4、heartbeatServices: 提供心跳所需的通盘工作。这包括创建心跳吸收器和心跳发送者。 * 5、metricRegistry: 追踪通盘已注册的 Metric,它当作联结 MetricGroup 和 MetricReporter * 6、archivedExecutionGraphStore: 存储奉行图ExecutionGraph的可序列化体式。 */ initializeServices(configuration, pluginManager); // 创建 DispatcherResourceManagerComponentFactory, 运行化各式组件的 工场实例 // 其实里面包含了三个贫苦的成员变量: // 创建 ResourceManager 的工场实例 // 创建 Dispatcher 的工场实例 // 创建 WebMonitorEndpoint 的工场实例 createDispatcherResourceManagerComponentFactory(configuration); // 创建 集群运行需要的一些组件:Dispatcher, ResourceManager 等 // 创 建 ResourceManager // 创 建 Dispatcher // 创 建 WebMonitorEndpoint clusterComponent = dispatcherResourceManagerComponentFactory.create(...)1. initializeServices():运行化各式工作
// 初 始 化 和 启 动 AkkaRpcService, 内 部 其 实 包 装 了 一 个 ActorSystem commonRpcService = AkkaRpcServiceUtils.createRemoteRpcService(...) // 运行化一个厚爱 IO 的线程池 ioExecutor = Executors.newFixedThreadPool(...) // 运行化 HA 工作组件,厚爱 HA 工作的是:ZooKeeperHaServices haServices = createHaServices(configuration, ioExecutor); // 运行化 BlobServer 工作端 blobServer = new BlobServer(configuration, haServices.createBlobStore()); blobServer.start(); // 运行化心跳工作组件, heartbeatServices = HeartbeatServices heartbeatServices = createHeartbeatServices(configuration); // 运行化一个用来存储 ExecutionGraph 的 Store, 已毕是: FileArchivedExecutionGraphStore archivedExecutionGraphStore = createSerializableExecutionGraphStore(...)2. createDispatcherResourceManagerComponentFactory(configuration)运行化了多组件的工场实例
1、DispatcherRunnerFactory,默许已毕:DefaultDispatcherRunnerFactory 2、ResourceManagerFactory,默许已毕:StandaloneResourceManagerFactory 3、RestEndpointFactory,默许已毕:SessionRestEndpointFactory clusterComponent = dispatcherResourceManagerComponentFactory .create(configuration, ioExecutor, commonRpcService, haServices, blobServer, heartbeatServices, metricRegistry, archivedExecutionGraphStore, new RpcMetricQueryServiceRetriever(metricRegistry.getMetricQueryServiceRpcService()), this);3. 创建 WebMonitorEndpoint
/************************************************* * 创建 WebMonitorEndpoint 实例, 在 Standalone 步地下:DispatcherRestEndpoint * 1、restEndpointFactory = SessionRestEndpointFactory * 2、webMonitorEndpoint = DispatcherRestEndpoint * 3、highAvailabilityServices.getClusterRestEndpointLeaderElectionService() = ZooKeeperLeaderElectionService * 面前这个 DispatcherRestEndpoint 的作用是: * 1、运行化的过程中,会一大堆的 Handler * 2、启动一个 Netty 的工作端,绑定了这些 Handler * 3、当 client 通过 flink 敕令奉行了某些操作(发起 restful 请求), 工作端由 webMonitorEndpoint 来奉行处理 * 4、举个例子: 要是通过 flink run 提交一个 Job,那么临了是由 webMonitorEndpoint 中的 JobSubmitHandler 来奉行处理 * 5、补充一个:job 由 JobSubmitHandler 奉行完了之后,转交给 Dispatcher 去退换奉行 */ webMonitorEndpoint = restEndpointFactory.createRestEndpoint( configuration, dispatcherGatewayRetriever, resourceManagerGatewayRetriever, blobServer, executor, metricFetcher, highAvailabilityServices.getClusterRestEndpointLeaderElectionService(), fatalErrorHandler );4. 创建 resourceManager
/************************************************* * 创建 StandaloneResourceManager 实例对象 * 1、resourceManager = StandaloneResourceManager * 2、resourceManagerFactory = StandaloneResourceManagerFactory */ resourceManager = resourceManagerFactory.createResourceManager( configuration, ResourceID.generate(), rpcService, highAvailabilityServices, heartbeatServices, fatalErrorHandler, new ClusterInformation(hostname, blobServer.getPort()), webMonitorEndpoint.getRestBaseUrl(), metricRegistry, hostname );
protected ResourceManager<ResourceID> createResourceManager( Configuration configuration, ResourceID resourceId, RpcService rpcService, HighAvailabilityServices highAvailabilityServices, HeartbeatServices heartbeatServices, FatalErrorHandler fatalErrorHandler, ClusterInformation clusterInformation, @Nullable String webInterfaceUrl, ResourceManagerMetricGroup resourceManagerMetricGroup, ResourceManagerRuntimeServices resourceManagerRuntimeServices) { final Time standaloneClusterStartupPeriodTime = ConfigurationUtils.getStandaloneClusterStartupPeriodTime(configuration); /************************************************* * 致密: 得到一个 StandaloneResourceManager 实例对象 */ return new StandaloneResourceManager( rpcService, resourceId, highAvailabilityServices, heartbeatServices, resourceManagerRuntimeServices.getSlotManager(), ResourceManagerPartitionTrackerImpl::new, resourceManagerRuntimeServices.getJobLeaderIdService(), clusterInformation, fatalErrorHandler, resourceManagerMetricGroup, standaloneClusterStartupPeriodTime, AkkaUtils.getTimeoutAsTime(configuration) ); }
/** requestSlot():秉承 solt请求 sendSlotReport(..): 将solt请求发送TaskManager registerJobManager(...): 注册job处分者。 该job指的是 提交给flink的诈骗步骤 registerTaskExecutor(...): 注册task奉行者。 **/ public ResourceManager(RpcService rpcService, ResourceID resourceId, HighAvailabilityServices highAvailabilityServices, HeartbeatServices heartbeatServices, SlotManager slotManager, ResourceManagerPartitionTrackerFactory clusterPartitionTrackerFactory, JobLeaderIdService jobLeaderIdService, ClusterInformation clusterInformation, FatalErrorHandler fatalErrorHandler, ResourceManagerMetricGroup resourceManagerMetricGroup, Time rpcTimeout) { /************************************************* * 致密: 当奉行完了这个构造步骤的期间,会触发调用 onStart() 步骤奉行 */ super(rpcService, AkkaRpcServiceUtils.createRandomName(RESOURCE_MANAGER_NAME), null);
protected RpcEndpoint(final RpcService rpcService, final String endpointId) { this.rpcService = checkNotNull(rpcService, "rpcService"); this.endpointId = checkNotNull(endpointId, "endpointId"); /************************************************* * 致密:ResourceManager 或者 TaskExecutor 中的 RpcServer 已毕 * 以 ResourceManager 为例评释: * 启动 ResourceManager 的 RPCServer 工作 * 这里启动的是 ResourceManager 的 Rpc 工作端。 * 吸收 TaskManager 启动好了而之后, 进行注册和心跳,来文告 Taskmanagaer 的资源情况 * 通过动态代理的体式构建了一个Server */ this.rpcServer = rpcService.startServer(this);5. 在创建resourceManager同级:启动任务吸收器Starting Dispatcher
/************************************************* * 创建 并启动 Dispatcher * 1、dispatcherRunner = DispatcherRunnerLeaderElectionLifecycleManager * 2、dispatcherRunnerFactory = DefaultDispatcherRunnerFactory * 第一个参数:ZooKeeperLeaderElectionService * - * 老版块: 这个方位是奏凯创建一个 Dispatcher 对象然后调用 dispatcher.start() 来启动 * 新版块: 奏凯创建一个 DispatcherRunner, 里面即是要创建和启动 Dispatcher * - * DispatcherRunner 是对 Dispatcher 的封装。 * DispatcherRunner被创建的代码的里面,会创建 Dispatcher并启动 */ log.debug("Starting Dispatcher."); dispatcherRunner = dispatcherRunnerFactory.createDispatcherRunner( highAvailabilityServices.getDispatcherLeaderElectionService(), fatalErrorHandler, // TODO_ZYM 致密: 提防第三个参数 new HaServicesJobGraphStoreFactory(highAvailabilityServices), ioExecutor, rpcService, partialDispatcherServices );
Dispatcher 启动后,将会恭候任务提交,要是有任务提交,则会经过submitJob(...)函数插足后续处理。
提交(一个Flink诈骗的提交必须经过三个graph的蜕变)StreamGraph
是把柄用户通过 Stream API 编写的代码生成的起先的图。用来默示步骤的拓扑结构。不错用一个 DAG 来默示),DAG 的极点是 StreamNode,边是 StreamEdge,边包含了由哪个 StreamNode 依赖哪个 StreamNode。
StreamNode:用来代表 operator 的类,并具有通盘关联的属性,欧博博彩开户如并发度、入边和出边等。 StreamEdge:默示联结两个StreamNode的边。DataStream 上常见的 transformation 有 map、flatmap、filter等(见DataStream Transformation了解更多)。这些transformation会构造出一棵 StreamTransformation 树,通过这棵树蜕变成 StreamGraph
皇冠博彩平台受欢迎博彩平台之一,拥有多样化博彩游戏赛事直播,博彩攻略技巧分享,您博彩游戏中享受乐趣收益。平台安全稳定,操作简便,充值提款便捷,您提供最佳博彩体验最高博彩收益。以map步骤为例,望望源码
public <R> SingleOutputStreamOperator<R> map(MapFunction<T, R> mapper) { // 通过java reflection抽出mapper的复返值类型 TypeInformation<R> outType = TypeExtractor.getMapReturnTypes(clean(mapper), getType(), Utils.getCallLocationName(), true); // 复返一个新的DataStream,SteramMap 为 StreamOperator 的已毕类 return transform("Map", outType, new StreamMap<>(clean(mapper))); } public <R> SingleOutputStreamOperator<R> transform(String operatorName, TypeInformation<R> outTypeInfo, OneInputStreamOperator<T, R> operator) { // read the output type of the input Transform to coax out errors about MissingTypeInfo transformation.getOutputType(); // 新的transformation会联结上头前DataStream中的transformation,从而构建成一棵树 OneInputTransformation<T, R> resultTransform = new OneInputTransformation<>( this.transformation, operatorName, operator, outTypeInfo, environment.getParallelism()); @SuppressWarnings({ "unchecked", "rawtypes" }) SingleOutputStreamOperator<R> returnStream = new SingleOutputStreamOperator(environment, resultTransform); // 通盘的transformation皆会存到 env 中,调用execute时遍历该list生成StreamGraph getExecutionEnvironment().addOperator(resultTransform); return returnStream; }
map蜕变将用户自界说的函数MapFunction包装到StreamMap这个Operator中,再将StreamMap包装到OneInputTransformation,临了该transformation存到env中,当调用env.execute时,遍历其中的transformation纠合构造出StreamGraph
JobGraph
(1) StreamGraph经过优化青年景了 JobGraph,提交给 JobManager 的数据结构。主要的优化为,将多个得当要求的节点 chain 在总计当作一个节点。
(2) JobGraph 用来由 JobClient 提交给 JobManager,是由极点(JobVertex)、中间效用(IntermediateDataSet)和边(JobEdge)构成的 DAG 图。
(3) JobGraph 界说功课级别的竖立,而每个极点和中间效用界说具体操作和中间数据的竖立。
JobVertex
JobVertex 非常于是 JobGraph 的极点。经过优化后得当要求的多个StreamNode可能会chain在总计生成一个JobVertex,即一个JobVertex包含一个或多个operator,JobVertex的输入是JobEdge,输出是IntermediateDataSet。
IntermediateDataSet
JobVertex的输出,即经过operator处理产生的数据集。
皇冠体育
JobEdge
job graph中的一条数据传输通说念。source 是IntermediateDataSet,sink 是 JobVertex。即数据通过JobEdge由IntermediateDataSet传递给策画JobVertex。
(1) 起先是通过API会生成transformations,通过transformations会生成StreamGraph。
(2)将StreamGraph的某些StreamNode Chain在总计生成JobGraph,前两步蜕变皆是在客户端完成。
(3)临了会将JobGraph蜕变为ExecutionGraph,比拟JobGraph会增多并行度的认识,这一步是在Jobmanager里完成。
ExecutionJobVertex
ExecutionJobVertex逐个双应JobGraph中的JobVertex
ExecutionVertex
一个ExecutionJobVertex对应n个ExecutionVertex,其中n即是算子的并行度。ExecutionVertex即是并行任务的一个子任务
Execution
皇冠信用盘口Execution 是对 ExecutionVertex 的一次奉行,通过 ExecutionAttemptId 来独一标志。
IntermediateResult
在 JobGraph 顶用 IntermediateDataSet 默示 JobVertex 的对外输出,一个 JobGraph 可能有 n(n >=0) 个输出。在 ExecutionGraph 中,与此对应的即是 IntermediateResult。每一个 IntermediateResult 就有 numParallelProducers(并行度) 个分娩者,每个分娩者的在相应的 IntermediateResult 上的输出对应一个 IntermediateResultPartition。IntermediateResultPartition 默示的是 ExecutionVertex 的一个输出分区
ExecutionEdge
ExecutionEdge 默示 ExecutionVertex 的输入,通过 ExecutionEdge 将 ExecutionVertex 和 IntermediateResultPartition 联结起来,进而在不同的 ExecutionVertex 之间修复臆想。
ExecutionGraph的构建
构建JobInformation 构建ExecutionGraph 将JobGraph进行拓扑排序,得回sortedTopology极点纠合// ExecutionGraphBuilder public static ExecutionGraph buildGraph( @Nullable ExecutionGraph prior, JobGraph jobGraph, ...) throws JobExecutionException, JobException { // 构建JobInformation // 构建ExecutionGraph // 将JobGraph进行拓扑排序,得回sortedTopology极点纠合 List<JobVertex> sortedTopology = jobGraph.getVerticesSortedTopologicallyFromSources(); executionGraph.attachJobGraph(sortedTopology); return executionGraph; }
构建ExecutionJobVertex,联结IntermediateResultPartition和ExecutionVertex
//ExecutionGraph public void attachJobGraph(List<JobVertex> topologiallySorted) throws JobException { for (JobVertex jobVertex : topologiallySorted) { // 构建ExecutionJobVertex ExecutionJobVertex ejv = new ExecutionJobVertex( this, jobVertex, 1, maxPriorAttemptsHistoryLength, rpcTimeout, globalModVersion, createTimestamp); // 联结IntermediateResultPartition和ExecutionVertex ev.connectToPredecessors(this.intermediateResults); } // ExecutionJobVertex public void connectToPredecessors(Map<IntermediateDataSetID, IntermediateResult> intermediateDataSets) throws JobException { List<JobEdge> inputs = jobVertex.getInputs(); for (int num = 0; num < inputs.size(); num++) { JobEdge edge = inputs.get(num); IntermediateResult ires = intermediateDataSets.get(edge.getSourceId()); this.inputs.add(ires); int consumerIndex = ires.registerConsumer(); for (int i = 0; i < parallelism; i++) { ExecutionVertex ev = taskVertices[i]; ev.connectSource(num, ires, edge, consumerIndex); } } }
拆分权略(可奉行才智)
// ExecutionVertex public void connectSource(int inputNumber, IntermediateResult source, JobEdge edge, int consumerNumber) { final DistributionPattern pattern = edge.getDistributionPattern(); final IntermediateResultPartition[] sourcePartitions = source.getPartitions(); ExecutionEdge[] edges; switch (pattern) { // 卑鄙 JobVertex 的输入 partition 算法,要是是 forward 或 rescale 的话为 POINTWISE case POINTWISE: edges = connectPointwise(sourcePartitions, inputNumber); break; // 每一个并行的ExecutionVertex节点皆会联结到源节点产生的通盘中间效用IntermediateResultPartition case ALL_TO_ALL: edges = connectAllToAll(sourcePartitions, inputNumber); break; default: throw new RuntimeException("Unrecognized distribution pattern."); } inputEdges[inputNumber] = edges; for (ExecutionEdge ee : edges) { ee.getSource().addConsumer(ee, consumerNumber); } } private ExecutionEdge[] connectPointwise(IntermediateResultPartition[] sourcePartitions, int inputNumber) { final int numSources = sourcePartitions.length; final int parallelism = getTotalNumberOfParallelSubtasks(); // 要是并发数等于partition数,则一双一进行联结 if (numSources == parallelism) { return new ExecutionEdge[] { new ExecutionEdge(sourcePartitions[subTaskIndex], this, inputNumber) }; } // 要是并发数大于partition数,则一双多进行联结 else if (numSources < parallelism) { int sourcePartition; if (parallelism % numSources == 0) { int factor = parallelism / numSources; sourcePartition = subTaskIndex / factor; } else { float factor = ((float) parallelism) / numSources; sourcePartition = (int) (subTaskIndex / factor); } return new ExecutionEdge[] { new ExecutionEdge(sourcePartitions[sourcePartition], this, inputNumber) }; } // 果并发数小于partition数,则多对一进行联结 else { if (numSources % parallelism == 0) { int factor = numSources / parallelism; int startIndex = subTaskIndex * factor; ExecutionEdge[] edges = new ExecutionEdge[factor]; for (int i = 0; i < factor; i++) { edges[i] = new ExecutionEdge(sourcePartitions[startIndex + i], this, inputNumber); } return edges; } else { float factor = ((float) numSources) / parallelism; int start = (int) (subTaskIndex * factor); int end = (subTaskIndex == getTotalNumberOfParallelSubtasks() - 1) ? sourcePartitions.length : (int) ((subTaskIndex + 1) * factor); ExecutionEdge[] edges = new ExecutionEdge[end - start]; for (int i = 0; i < edges.length; i++) { edges[i] = new ExecutionEdge(sourcePartitions[start + i], this, inputNumber); } return edges; } } } private ExecutionEdge[] connectAllToAll(IntermediateResultPartition[] sourcePartitions, int inputNumber) { ExecutionEdge[] edges = new ExecutionEdge[sourcePartitions.length]; for (int i = 0; i < sourcePartitions.length; i++) { IntermediateResultPartition irp = sourcePartitions[i]; edges[i] = new ExecutionEdge(irp, this, inputNumber); } return edges; }
复返ExecutionGraph
TaskManagerTaskManager启动
public static void runTaskManager(Configuration configuration, ResourceID resourceId) throws Exception { //主要运行化一堆的service,并新建一个org.apache.flink.runtime.taskexecutor.TaskExecutor final TaskManagerRunner taskManagerRunner = new TaskManagerRunner(configuration,resourceId); //调用TaskExecutor的start()步骤 taskManagerRunner.start(); }
TaskExecutor :submitTask()
接着的贫苦函数是shumitTask()函数,该函数默契过AKKA机制,向TaskManager发出一个submitTask的音信请求,TaskManager收到音信请求后,会奉行submitTask()步骤。(不祥了部分代码)。
威尼斯人娱乐public CompletableFuture<Acknowledge> submitTask( TaskDeploymentDescriptor tdd, JobMasterId jobMasterId, Time timeout) { jobInformation = tdd.getSerializedJobInformation().deserializeValue(getClass().getClassLoader()); taskInformation = tdd.getSerializedTaskInformation().deserializeValue(getClass().getClassLoader()); TaskMetricGroup taskMetricGroup = taskManagerMetricGroup.addTaskForJob(xxx); InputSplitProvider inputSplitProvider = new RpcInputSplitProvider(xxx); TaskManagerActions taskManagerActions = jobManagerConnection.getTaskManagerActions(); CheckpointResponder checkpointResponder = jobManagerConnection.getCheckpointResponder(); LibraryCacheManager libraryCache = jobManagerConnection.getLibraryCacheManager(); ResultPartitionConsumableNotifier resultPartitionConsumableNotifier = jobManagerConnection.getResultPartitionConsumableNotifier(); PartitionProducerStateChecker partitionStateChecker = jobManagerConnection.getPartitionStateChecker(); final TaskLocalStateStore localStateStore = localStateStoresManager.localStateStoreForSubtask( jobId, tdd.getAllocationId(), taskInformation.getJobVertexId(), tdd.getSubtaskIndex()); final JobManagerTaskRestore taskRestore = tdd.getTaskRestore(); final TaskStateManager taskStateManager = new TaskStateManagerImpl( jobId, tdd.getExecutionAttemptId(), localStateStore, taskRestore, checkpointResponder); //新建一个Task Task task = new Task(xxxx); log.info("Received task {}.", task.getTaskInfo().getTaskNameWithSubtasks()); boolean taskAdded; try { taskAdded = taskSlotTable.addTask(task); } catch (SlotNotFoundException | SlotNotActiveException e) { throw new TaskSubmissionException("Could not submit task.", e); } if (taskAdded) { //启动任务 task.startTaskThread(); return CompletableFuture.completedFuture(Acknowledge.get()); }
临了创建奉行Task的线程,然后调用startTaskThread()来启动具体的奉行线程,Task线程里面的run()步骤承载了被奉行的中枢逻辑。
Task是奉行在TaskExecutor程度里的一个线程,底下来望望其run步骤
(1) 检测面前气象,平方情况为CREATED,要是是FAILED或CANCELING奏凯复返,其余气象将抛极端。
(2) 读取DistributedCache文献。
(3) 启动ResultPartitionWriter和InputGate。
(4) 向taskEventDispatcher注册partitionWriter。
(5) 把柄nameOfInvokableClass加载对应的类并实例化。
(6) 将气象置为RUNNING并奉行invoke步骤。
public void run() { while (true) { ExecutionState current = this.executionState; invokable = loadAndInstantiateInvokable(userCodeClassLoader, nameOfInvokableClass); network.registerTask(this); Environment env = new RuntimeEnvironment(. . . . ); invokable.setEnvironment(env); // actual task core work if (!transitionState(ExecutionState.DEPLOYING, ExecutionState.RUNNING)) { } // notify everyone that we switched to running notifyObservers(ExecutionState.RUNNING, null); executingThread.setContextClassLoader(userCodeClassLoader); // run the invokable invokable.invoke(); if (transitionState(ExecutionState.RUNNING, ExecutionState.FINISHED)) { notifyObservers(ExecutionState.FINISHED, null); } Finally{ // free the network resources network.unregisterTask(this); // free memory resources if (invokable != null) { memoryManager.releaseAll(invokable); } libraryCache.unregisterTask(jobId, executionId); removeCachedFiles(distributedCacheEntries, fileCache);回来
合座的经由与架构可能三两张图或者一言半辞就不错勾画出画面,关联词背后源码的已毕是精深的。源码的复杂度和当初遐想框架的持狂感,咱们唯独思象。目下咱们仅仅站在巨东说念主的肩膀上去学习。
轻松玩赚本篇的主题是"Flink架构与奉行经由",作念下小结,Flink on Yarn的提交奉行经由:
1 Flink任务提交后,Client向HDFS上传Flink的Jar包和竖立。
2 向Yarn ResourceManager提交任务。
3 ResourceManager分派Container资源并奉告对应的NodeManager启动ApplicationMaster。
4 ApplicationMaster启动后加载Flink的Jar包和竖立构建环境。
5 启动JobManager之后ApplicationMaster向ResourceManager苦求资源启动TaskManager。
6 ResourceManager分派Container资源后,由ApplicationMaster奉告资源所在节点的NodeManager启动TaskManager。
7 NodeManager加载Flink的Jar包和竖立构建环境并启动TaskManager。
8 TaskManager启动后向JobManager发送心跳包,并恭候JobManager向其分派任务。
皇冠体育官方网站